Advanced Data Analytics: Techniques And Applications
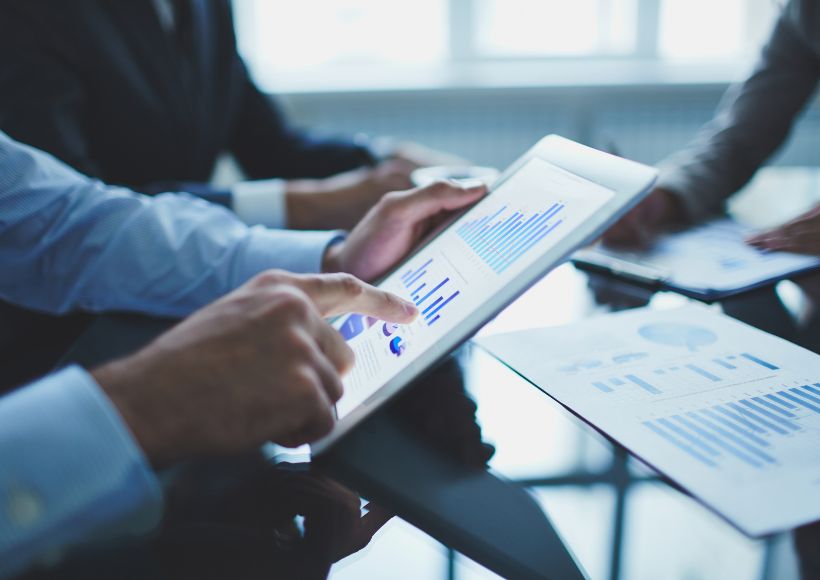
Advanced Data Analytics is the use of more advanced tools and techniques to explore unstructured data from complex datasets. It is more sophisticated than data analysis. Advanced Data Analytics enables organizations to identify deeper insights, facilitate quicker decision-making and improve performance in a more quantifiable manner.
In order to know what is advanced data analytics?, it can be helpful to understand the difference between advanced data analytics and basic data analysis. With basic (ordinary) analysis, we can analyze historical data for trends. Advanced analytics uses techniques like predictive modelling, machine learning, and artificial intelligence to identify potential trends in future periods and automate decision-making processes.
Generally, advanced data analytics allows organizations to progress from “what happened” to “what is going to happen” to “what we should do next.”
Table of Contents
Techniques Used in Advanced Data Analytics
Predictive analytics
In predicting the future, predictive analytics applies statistical models and machine learning methods. It is often used in finance, marketing, and supply chain.
Prescriptive analytics
This technique not only predicts the future but also suggests actions. Prescriptive analytics uses simulation and optimization methods in their recommendation.
Data mining
Data mining is the process of searching large data sets in order to discover patterns and relationships. It allows organizations to identify risks and find opportunities that are not detectable otherwise.
Machine Learning
Algorithms learn from data and improve over time. It’s used in fraud detection, customer segmentation, and product recommendations.
Natural Language Processing (NLP)
NLP allows machines to understand and process human language. It is useful for sentiment analysis and customer service automation.
Applications in Business
Businesses use advanced data analytics in various ways:
- Marketing: Personalizing content and targeting ads more accurately.
- Finance: Detecting fraud and managing risks.
- Retail: Optimizing inventory and pricing.
- Healthcare: Predicting patient outcomes and improving diagnosis.
- Manufacturing: Reducing downtime and improving quality control.
These examples show how analytics can enable better decision-making across functions. Usually, firms reporting using analytics will also note they experience improved results, improved customer satisfaction, and lower costs.
Data Analytics vs Data Analysis
Understanding the difference between data analytics and data analysis is important. Data analysis is a simple process of collecting, cleaning, and interpreting data. At the same time, data analytics consists of a broader range of data methods and tools that can be used for simple and advanced applications.
In business, both are important and useful. However, advanced data analytics can help organizations create more value from their data because they can deliver predictive and prescriptive insights beyond just descriptive insights.
Also Read : Business Analytics vs. Data Analytics
Data Analytics vs Data Science
Data analytics is another common comparison. Data analytics and data science overlap but are not the same. Data science is a wider field encompassing data analytics, as well as coding (e.g., Python or R), statistical modelling, and designing data architecture. A data scientist makes models, while a data analyst works more directly with those models and interprets results that feed into decision-making.
In practice, both roles are equally important. Advanced data analytics is, however, the action layer: it takes raw data and data models into decisions and strategies.
What Are the Business Benefits of Advanced Data and Analytics?
Companies that use Advanced Data Analytics gain measurable benefits. The following benefits best illustrate this:
1. Faster Decision-Making
Advanced analytics gives real-time insights. Rather than waiting for reports, teams have live dashboards and predictive models they can act upon immediately, which helps remove the guesswork and enhances the response time of every department.
2. Improved Customer Experience
Businesses can use customer behaviour and feedback to create as personalized a service as possible, which increases satisfaction. All aspects of the service, such as recommendations, support, and communication, can be customized. Customers tend to remain loyal to businesses that provide individualized service.
3. Cost Reduction
Analysis determines where we are wasting time, money, or resources. It can help us to improve operations, reduce inefficiencies, and allocate budgets better. This results in reduced operational costs more directly without the output being reduced as well.
4. Risk Management
Advanced models recognize unusual patterns, which can help companies predict and potentially mitigate fraud, errors, and operational risks. This provides a layer of protection that is proactive in finance, logistics, cybersecurity, and compliance.
5. Revenue Growth
Data will highlight new opportunities for businesses—such as what to sell, where to sell, and how much to charge. Companies can target campaigns, launch product offerings quicker, and reach the correct audience, giving them more conversions and building sales.
6. Better Forecasting and Planning
Predictive analytics provides precise information on the future, be it demand, customer needs, or inventory requirements. Better planning facilitates avoidance of being overstocked, rolling out ineffective products, and managing responding gaps in resource requirements.
7. Competitive Advantage
Businesses leveraging advanced analytics agilely adapt to their environment quickly, innovating quickly and serving customers better than the competition. This data-led adaptability allows them to compete effectively and understand the rapidly changing market as they move ahead.
8. Smarter Product Development
Analytics helps determine what users want, what makes them mad, and where they might have opportunities. These factors contribute to developing better product features quicker and provide insight into what users in the market want.
These examples demonstrate why advanced data analytics is necessary for business growth in today’s marketplace. Businesses in the future are going to demand more from working with data to stay relevant. From decision-making as part of your daily operations to decisions relating to long-term strategy, working with data means better outcomes in general.
Conclusion
Advanced data analytics is transforming how organizations work. Using the right tools and methods will allow organizations to improve their understanding of their data and make informed strategic decisions faster. Whether you’re in healthcare, retail, or finance, and when appropriate, advanced analytics can identify new pathways for opportunity and avoid duplicated and unnecessary waste.
With definitions of what data analytics is and how it is related to data science and utilizing the right data analytics tools, companies can successfully begin to convert their data into an actual strategic asset.