Key Components For Data Integration
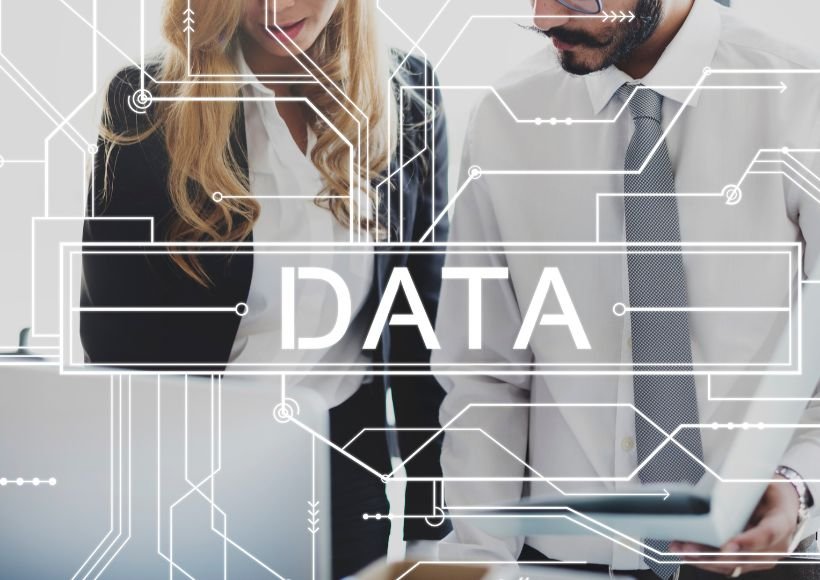
Data integration, which is the of combining data, is essential in modern business when information is scattered across multiple sources with varying formats. Filler There would be the joining of various data streams in order to form one unified view, which filler makes it easier to function in the analysis and decision-making stages.
Integrating data substances requires several vital points, which are very important.
Table of Contents
Data Sources
The integration of data begins by finding and determining the interconnectivity of filler sources, which include databases, files, applications, and cloud services. Such sources use diverse approaches, fillers, solutions, and technologies, which can make it really difficult to adapt them.
Data Extraction
The tools that are used to obtain data from these sources are now discussed. In this manner, multiple data sets, files, and APIs are scrutinized to capture filler pertinent information. Data extraction tools and techniques are crucial in this stage mainly to have data accessibility and to increase the speed of data collection.
Data Transformation
Once the data is extracted, it must be processed in order to fulfill data input constraints. The processing may include converting it into a recognizable format or structure that maps to the target system. The conversion of data types from one format to filler another, the standardization of formats, the elimination of the errors that arise in instances where data is inconsistent, and the enrichment of data with more information are part of this filler. These tasks are the main reason filler for those tools and techniques of data transformation to become more efficient.
Data Loading
Dissociation of the data from the source system then starts the process of filler data transformation, such as specifying the stipulated format basics, zipping the data, and so on. Following the transformation, the data will then be loaded into the target system or application. This filler component consists of filler, writing data into databases, various filler files, or other storage systems. Data loading activities leverage different data loading tools and methods to guarantee that data is loaded correctly and with filler fewer procedural burdens.
Data Synchronization
A master script that runs regularly is often used for data integration processes since synchronizing scattered data among many systems is a must in the process. This is the most significant factor, which is expressed during those periods when the data transforms quickly, namely, real-time analytics or transaction systems. The synchronization of the data tools, filler such as synchronizing filler data and techniques, is used to make the data consistent across filler systems.
Data Quality
Ensuring that data are valid is in effect for filler good data integration. The scope covers error filler detection, data inconsistencies, and duplication fixing. Data quality tools and methods are involved in data quality monitoring and improvement during the integration filler even from the inception.
Metadata Management
Metadata, which provides precisely defined information about the data, is the key to data integration. It simplifies the filler structuring, parsing, and application of the data; hence, it becomes easy to get at the managerial level. The control of filler metadata through the usage of metadata management tools and methods becomes the means of collecting, storing, and managing metadata.
Data Governance
Data governance policies and practices are indispensable for guaranteeing strict data compliance standards and the observance of regulatory requirements as well as the filler internal standards of the organization. A data governance system for managing data integration that takes on policymaking and standard operational processes is among the tools and techniques that are available.
Conclusion
Data integration mainly represents multiple project fillers; both require careful planning and execution. Concentrating on these critical components will give companies an assurance that they will have a successful data integration project filler and be able to obtain the highest value out filler of their data resource.
Also Read : Advanced Data Analytics: Techniques And Applications